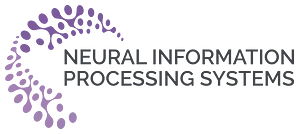
II. Transfer Fair Decision-Making Under Distribution Shif
B. An, Z. Che, M. Ding, and F. Huang, “Transferring Fairness under Distribution Shifts via Fair Consistency Regularization”, Neural Information Processing System (NeurIPS), 2022. Paper Link, Code Link & Presentation Link.
The increasing reliance on ML models in high-stakes tasks has raised a major concern on fairness violations. Although there has been a surge of work that improves algorithmic fairness, most of them are under the assumption of an identical training and test distribution. In many real-world applications, however, such an assumption is often violated as previously trained fair models are often deployed in a different environment, and the fairness of such models has been observed to collapse. In this paper, we study how to transfer model fairness under distribution shifts, a widespread issue in practice. We conduct a fine-grained analysis of how the fair model is affected under different types of distribution shifts and find that domain shifts are more challenging than subpopulation shifts. Inspired by the success of self-training in transferring accuracy under domain shifts, we derive a sufficient condition for transferring group fairness. Guided by it, we propose a practical algorithm with a fair consistency regularization as the key component. A synthetic dataset benchmark, which covers all types of distribution shifts, is deployed for experimental verification of the theoretical findings. Experiments on synthetic and real datasets including image and tabular data demonstrate that our approach effectively transfers fairness and accuracy under various distribution shifts.
Algorithmic fairness of a model is likely to collapse when encountering a distribution shift [1,2].
[1] Ding, Frances, et al. “Retiring adult: New datasets for fair machine learning.” Advances in Neural Information Processing Systems 34 (2021): 6478-6490.
[2] Schrouff, Jessica, et al. “Maintaining fairness across distribution shift: do we have viable solutions for real-world applications?.” arXiv preprint arXiv:2202.01034 (2022).
How to transfer fairness in machine learning models?
The answer is: to minimize & balance consistency loss across groups
- Mild Assumptions on Data:
- Two domains share the same underlying data generation process.
- Intra-group expansion (every group is self-connected with certain transformations).
- Separation.
- Claim 1: fairness in source domain + same level of invariance across groups —> fairness in target domain
- Supporting evidence: We bound unfairness via the worst-group consistency loss
- Claim 2: Accuracy in source domain + invariance to data transformations —> accuracy in target domain
- Supporting evidence: [Wei et al. 2021]
Experimental Results
- Experiments on Controlled Data Distribution Shifts
![]()
- Our method can transfer fairness and accuracy under various types of distribution shift.
- Experiments on Real-World Image Data
![]()
- Our method achieves the best Pareto frontiers.
- Experiments on Tabular Data
![]()
- Our method achieves better fairness in most of states.
- Absent of powerful transformations for tabular data limits the performance of our method.
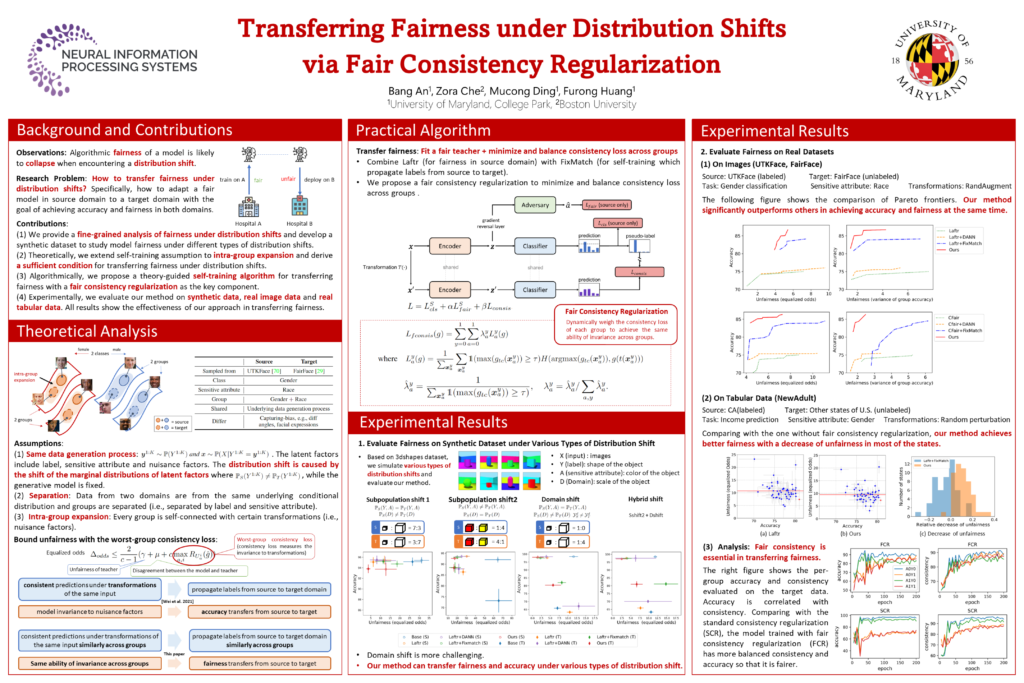