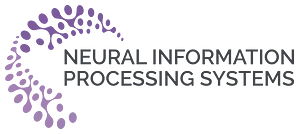
IV. Autonomous Data Augmentation Without Domain Knowledge (with no candidate types of augmentations to start with)
K. Yang, Y. Sun, J. Su, F. He, X. Tian, F. Huang, T. Zhou, D. Tao, “Adversarial Auto- Augment with Label Preservation: A Representation Learning Principle Guided Approach”, Neural Information Processing System (NeurIPS), 2022. Paper Link, Code Link & Presentation Link.
Data augmentation is a critical contributing factor to the success of deep learning but heavily relies on prior domain knowledge which is not always available. Recent works on automatic data augmentation learn a policy to form a sequence of augmentation operations, which are still pre-defined and restricted to limited options. In this paper, we show that a prior-free autonomous data augmentation’s objective can be derived from a representation learning principle that aims to preserve the minimum sufficient information of the labels. Given an example, the objective aims at creating a distant “hard positive example” as the augmentation, while still preserving the original label. We then propose a practical surrogate to the objective that can be optimized efficiently and integrated seamlessly into existing methods for a broad class of machine learning tasks, e.g., supervised, semi-supervised, and noisy-label learning. Unlike previous works, our method does not require training an extra generative model but instead leverages the intermediate layer representations of the end-task model for generating data augmentations. In experiments, we show that our method consistently brings non-trivial improvements to the three aforementioned learning tasks from both efficiency and final performance, either or not combined with strong pre-defined augmentations, e.g., on medical images when domain knowledge is unavailable and the existing augmentation techniques perform poorly.
Problem with Current Data Augmentations
- They are based on pre-defined operations and are not fully automated.
- They rely on domain knowledge to preserve label and are thus restricted to few domains.
Proper Data Augmentation Leads to Optimal Representation
Our solution: Label-Preserving Adversarial Auto-Augment (LPA3)
Experiments
In experiments, our method brings non-trivial efficiency and final performance improvements, as a highlight, even on medical images when domain knowledge is unavailable and the existing augmentation techniques perform poorly.
- Visualization on MedMNIST
- Semi-supervised learning
- Noisy-label learning
- Medical image classification
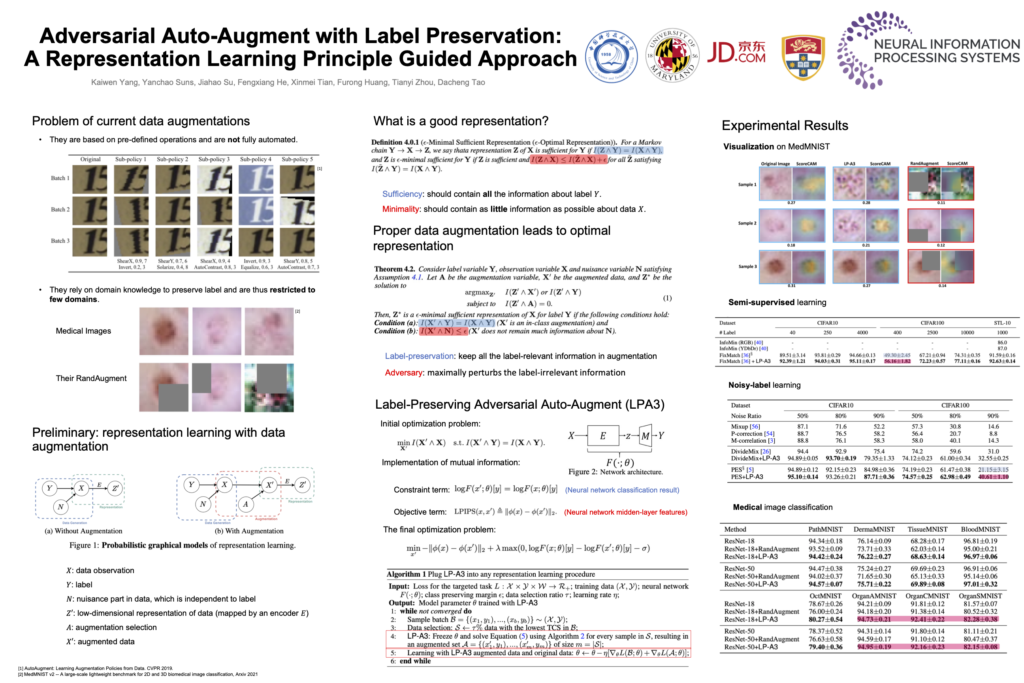